By Stephen DeAngelis
Decision-making is an important part of life — regardless of whether the decisions are personal or work-related. In the Digital Age, decisionmakers in the business world have been encouraged to rely more on data-driven decisions than on personal intuition. Sounds like good advice. There is a downside to such advice — data can mislead. The problem is not with the data per se. The problem lies in how that data is analyzed and reported. Sema K. Sgaier, CEO and Co-Founder of Surgo Health, and her colleagues Vincent Huang and Grace Charles explain, “Much of artificial intelligence (AI) in common use is dedicated to predicting people’s behavior. It tries to anticipate your next purchase, your next mouse-click, your next job move. But such techniques can run into problems when they are used to analyze data for health and development programs. If we do not know the root causes of behavior, we could easily make poor decisions and support ineffective and prejudicial policies.”[1]
The analysis challenge described above is often discussed in terms of causality versus correlation. Finding causation is always more important than simply discovering correlations. Correlations can easily lead one to believe something that is not true. To highlight that point, Tyler Vigen, a Partner at Boston Consulting Group (BCG), started a site, when he was a student at Harvard University, called Spurious Correlations. Vigen has shown, for example, that there is an annual correlation between the number of people who have drowned by falling into a swimming pool and the number of films in which Nicolas Cage has appeared and that the divorce rate in Maine correlates to the per capita consumption of margarine in the United States. Clearly, making decisions based on such correlations would be unwise.
A few years ago, European academics Joris M. Mooij, Jonas Peters, Dominik Janzing, Jakob Zscheischler, and Bernhard Schölkopf wrote, “An advantage of having knowledge about causal relationships rather than about statistical associations is that the former enables prediction of the effects of actions that perturb the observed system. While the gold standard for identifying causal relationships is controlled experimentation, in many cases, the required experiments are too expensive, unethical, or technically impossible to perform. The development of methods to identify causal relationships from purely observational data therefore constitutes an important field of research.”[2] That field of research has come to be known as Causal AI.
The Importance of Causal AI
Sgaier and her associates note, “Being able to predict an outcome is not the same as understanding what actually causes it. … [Causal AI] can open up the black box within which purely predictive models of AI operate. Causal AI can move beyond correlation to highlight the precise relationships between causes and effects.” The staff at causaLens goes even further. They insist, “Causal AI is the only technology that can reason and make choices like humans do. It utilizes causality to go beyond narrow machine learning predictions and can be directly integrated into human decision-making. It is the only AI system organizations can trust with their biggest challenges — a revolution in enterprise AI.”[3]
Gaurav Shekhar, Associate Director of Data Science at Fidelity International, notes that much of the desired analysis companies want to perform relies heavily on causation. He writes, “New approaches to machine learning based on principles of causal reasoning provide us with a promising path forward. Causal inference bridges the gap between prediction and decision-making and allows researchers and program designers to simulate an intervention and infer causality by relying on already available data.”[4] To demonstrate his point he created the following graphic.
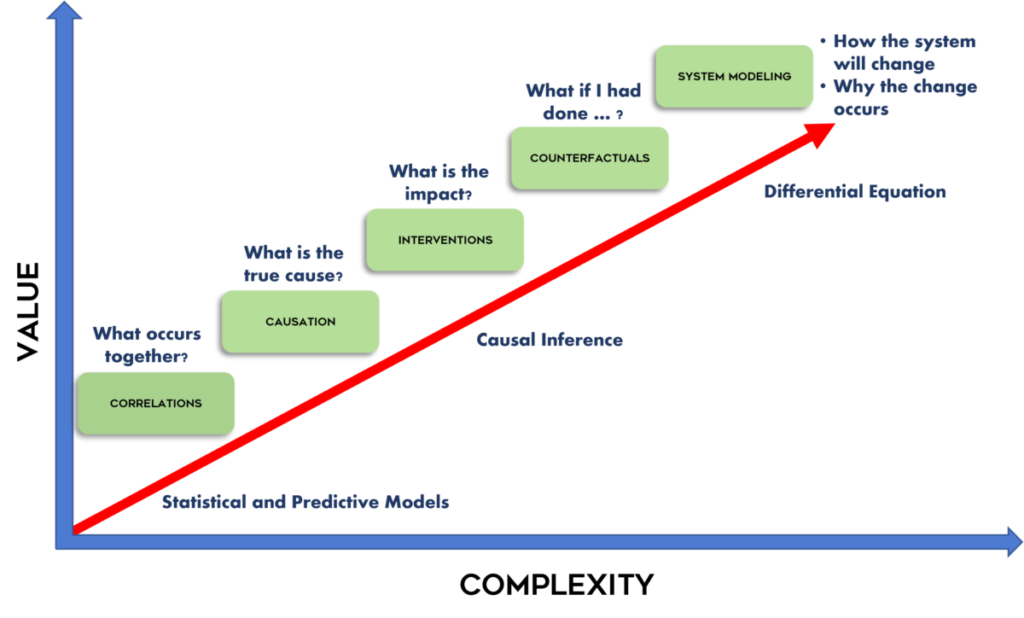
According to Shekhar, some of the key benefits of causal AI include: Enhanced model robustness; better measurement of intervention impacts; increased understanding of counterfactual (what-if) scenarios; and continued learning by the AI system. He elaborates, “Causal models remain robust when underlying data changes and thus can help in generalizing the solution to unseen data. Causal AI helps measure the impact of an intervention [which is a very important] tool for decision-making. Causal models also allow us to respond to situations we haven’t seen before and enable the solution to plan for unforeseen counterfactual situation. [Finally,] causal models allow humans to generalize previously gained knowledge to unseen and different challenges.” Tom Farrand, Director of Product at causaLens, adds, “Causal AI is powerful because it allows you to identify and eliminate spurious correlations using the existing observed data — without the need to run a controlled trial.”[5]
Concluding Thoughts
Sgaier and her colleagues conclude, “The field of causal AI is evolving rapidly. As its potential becomes more apparent, researchers are putting it to work in fields as diverse as climate change and health, demonstrating its broad potential. … We continually run up against the limits of what we are able to observe and the methods available to analyze our data. Causal AI is the next logical step, made feasible by recent technological transformations and the increasing pervasiveness of data. Its advantage over some other disciplines in the social sciences — and indeed over predictive AI — is that it can help identify the precise causal factors that directly lead to particular behaviors or outcomes, and it can efficiently test different approaches to changing those behaviors or outcomes.”
The staff at Rapidminer adds, “While AI can help businesses in a myriad of ways, traditional machine learning methods do have a few restrictions, including limited insights, narrow explainability, and a high susceptibility to bias. However, many of the intrinsic qualities of causal AI address these concerns and make causal AI a strong addition to any business’s data science toolkit.”[6]
Footnotes
[1] Sema K. Sgaier, Vincent Huang & Grace Charles, “The Case for Causal AI,” Stanford Social Innovation Review, Summer 2020.
[2] Joris M. Mooij, Jonas Peters, Dominik Janzing, Jakob Zscheischler, and Bernhard Schölkopf, “Distinguishing cause from effect using observational data: methods and benchmarks,” ArXiv, 11 December 2014.
[3] Staff, “Causal AI: The next generation of Enterprise AI,” causaLens.
[4] Gaurav Shekhar, “Causal AI — Enabling Data-Driven Decisions,” Towards Data Science, 26 May 2022.
[5] Tom Farrand, “A 6-Minute Introduction to Causal AI,” Towards Data Science, 19 August 2022.